New study demonstrates the potential for machine learning to accelerate the development of innovative drug delivery technologies
Scientists at the University of Toronto have successfully tested the use of machine learning models to guide the design of long-acting injectable drug formulations. The potential for machine learning algorithms to accelerate drug formulation could reduce the time and cost associated with drug development, making promising new medicines available faster.
The study was published today in Nature Communications and is one of the first to apply machine learning techniques to the design of polymeric long-acting injectable drug formulations.
The multidisciplinary research is led by Christine Allen from the University of Toronto’s department of pharmaceutical sciences and Alán Aspuru-Guzik, from the departments of chemistry and computer science. Both researchers are also members of the Acceleration Consortium, a global initiative that uses artificial intelligence and automation to accelerate the discovery of materials and molecules needed for a sustainable future.
“This study takes a critical step towards data-driven drug formulation development with an emphasis on long-acting injectables,” said Christine Allen, professor in pharmaceutical sciences at the Leslie Dan Faculty of Pharmacy, University of Toronto. “We’ve seen how machine learning has enabled incredible leap-step advances in the discovery of new molecules that have the potential to become medicines. We are now working to apply the same techniques to help us design better drug formulations and, ultimately, better medicines.”
“AI is transforming the way we do science. It helps accelerate discovery and optimization. This is a perfect example of a ‘Before AI’ and an ‘After AI’ moment and shows how drug delivery can be impacted by this multidisciplinary research”
Considered one of the most promising therapeutic strategies for the treatment of chronic diseases, long-acting injectables (LAI) are a class of advanced drug delivery systems that are designed to release their cargo over extended periods of time to achieve a prolonged therapeutic effect. This approach can help patients better adhere to their medication regimen, reduce side effects, and increase efficacy when injected close to the site of action in the body. However, achieving the optimal amount of drug release over the desired period of time requires the development and characterization of a wide array of formulation candidates through extensive and time-consuming experiments. This trial-and-error approach has created a significant bottleneck in LAI development compared to more conventional types of drug formulation.
“AI is transforming the way we do science. It helps accelerate discovery and optimization. This is a perfect example of a ‘Before AI’ and an ‘After AI’ moment and shows how drug delivery can be impacted by this multidisciplinary research,” said Alán Aspuru-Guzik, professor in chemistry and computer science, University of Toronto who also holds the CIFAR Artificial Intelligence Research Chair at the Vector Institute in Toronto.
From left: Zeqing Bao, PhD trainee in pharmaceutical sciences, and Riley Hickman, PhD trainee in chemistry, are co-authors on the study and supported study development and publication through data collection, results analysis, and preparation of the manuscript.
Making machine predictions useful to reduce ‘trial and error’ for new drug development
To investigate whether machine learning tools could accurately predict the rate of drug release, the research team trained and evaluated a series of eleven different models, including multiple linear regression (MLR), random forest (RF), light gradient boosting machine (lightGBM), and neural networks (NN). The data set used to train the selected panel of machine learning models was constructed from previously published studies by the authors and other research groups.
“Once we had the data set, we split it into two subsets: one used for training the models and one for testing. We then asked the models to predict the results of the test set and directly compared with previous experimental data. We found that the tree-based models, and specifically lightGBM, delivered the most accurate predictions,” said Pauric Bannigan, research associate with the Allen research group at the Leslie Dan Faculty of Pharmacy, University of Toronto.
As a next step, the team worked to apply these predictions and illustrate how machine learning models might be used to inform the design of new LAIs, the team used advanced analytical techniques to extract design criteria from the lightGBM model. This allowed the design of a new LAI formulation for a drug currently used to treat ovarian cancer. “Once you have a trained model, you can then work to interpret what the machine has learned and use that to develop design criteria for new systems,” said Bannigan. Once prepared, the drug release rate was tested and further validated the predictions made by the lightGBM model. “Sure enough, the formulation had the slow-release rate that we were looking for. This was significant because in the past it might have taken us several iterations to get to a release profile that looked like this, with machine learning we got there in one,” he said.
Expectations around the speed with which new drug formulations are developed have heightened drastically since the onset of the COVID-19 pandemic. “We’ve seen in the pandemic that there was a need to design a new formulation in weeks, to catch up with evolving variants. Allowing for new formulations to be developed in a short period of time, relative to what has been done in the past using conventional methods, is crucially important so that patients can benefit from new therapies,” said Allen explaining that the research team is also investigating using machine learning to support the development of novel mRNA and lipid nanoparticle formulations.
More robust databases needed for future advances
The results of the current study are encouraging and signal the potential for machine learning to reduce reliance on trial-and-error testing slowing the pace of development for long-acting injectables. However, the Allen and the research team identify that the lack of available open-source data sets in pharmaceutical sciences represents a significant challenge to future progress. “When we began this project, we were surprised by the lack of data reported across numerous studies using polymeric microparticles,” said Allen. “This meant the studies and the work that went into them couldn’t be leveraged to develop the machine learning models we need to propel advances in this space,” said Allen. “There is a real need to create robust databases in pharmaceutical sciences that are open access and available for all so that we can work together to advance the field,” she said.
"We want this to be the start of something and not the end of the story for machine learning in drug formulation."
To promote the move toward the accessible databases needed to support the integration of machine learning into pharmaceutical sciences more broadly, Allen and the research team have made their datasets and code and available on the open-source platform Zenodo.
“For this study our goal was to lower the barrier of entry to applying machine learning in pharmaceutical sciences,” said Bannigan. “We’ve made our data sets fully available so others can hopefully build on this work. We want this to be the start of something and not the end of the story for machine learning in drug formulation.”
More News
Image
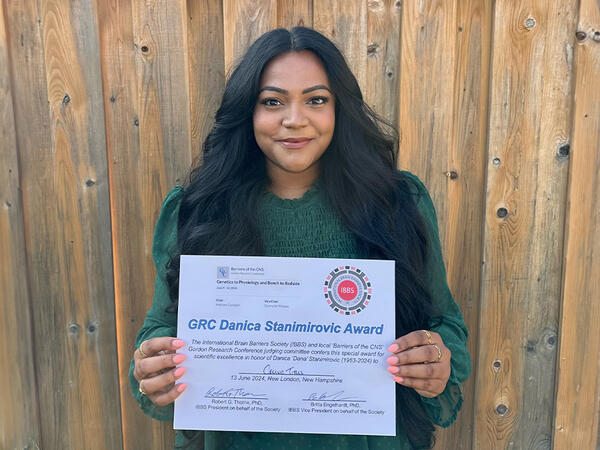
Faces of PharmSci: Celene Titus
PhD student Celene Titus is working in the field of HIV-Associated Neurocognitive Impairments (NCI) and brain inflammation to improve HIV patients' quality of life and deepen our understanding of brain inflammation in HIV infection.
Read More
Image
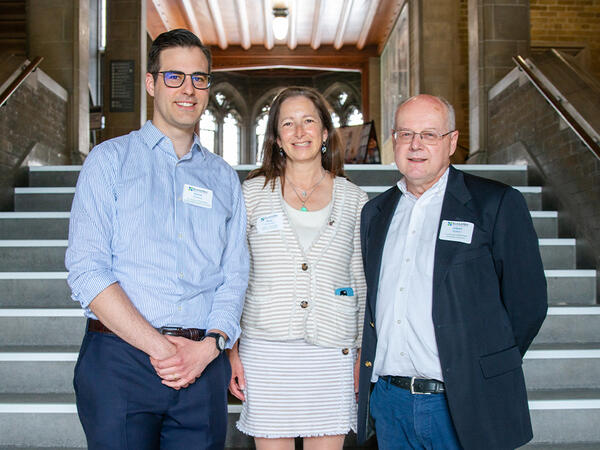
BioHubNet set to bridge academia and industry to strengthen Canada’s biomanufacturing talent pipeline
To kick-off the new launched Biomanufacturing Hub Network (BioHubNet) initiative, partners and collaborators met at U of T's Hart House to address key challenges in Canada's biomanufacturing sector.
Read More
Image
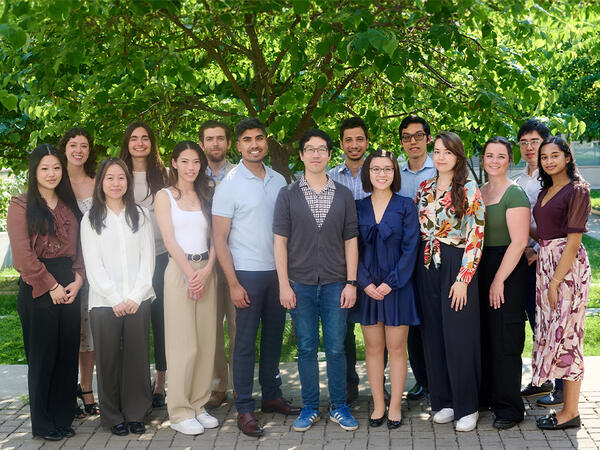
Exploring Tomorrow's Breakthroughs: GRIP 2024
Last week, the Leslie Dan Faculty of Pharmacy hosted its 2024 GRIP symposium, attended by 200 faculty, staff, and students, showcasing diverse graduate student research.
Read More